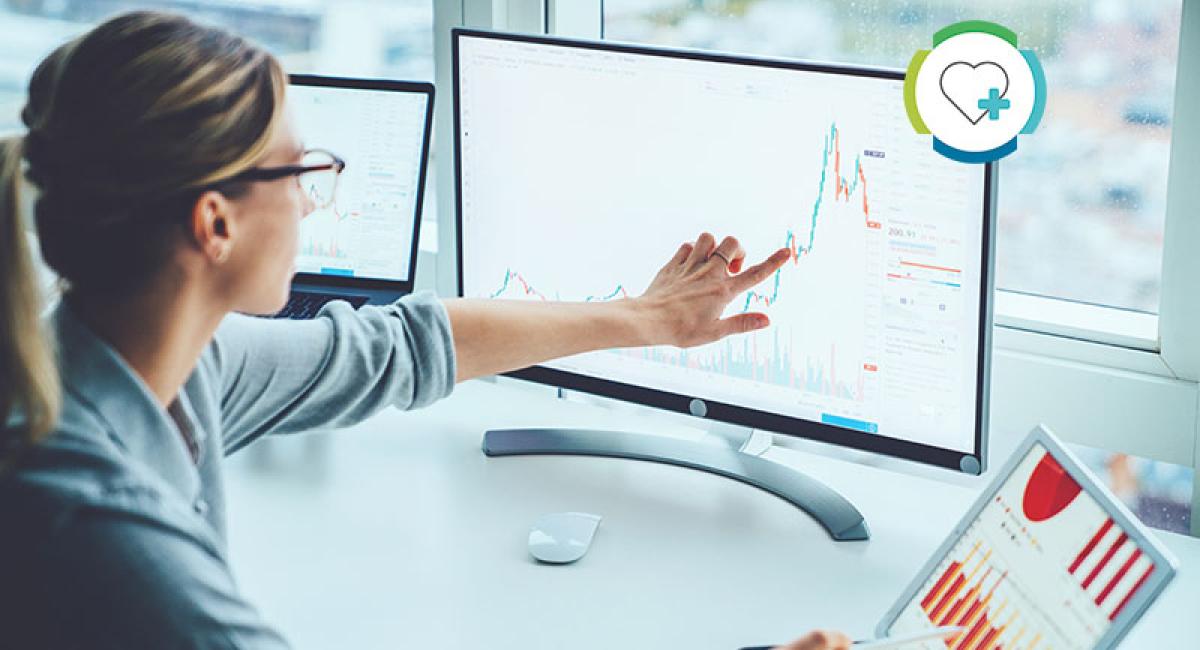
Machine learning enables earlier prediction of future heart failure in patients
Heart failure is a common condition with serious consequences; more than 20% of patients die within a year of diagnosis. Machine learning algorithms, used on data from GP electronic health records, allow us to predict in an early stage whether a patient is at increased risk of developing heart failure. This enables targeted preventive intervention, which may prevent or delay serious damage. This study was conducted by the Nivel in collaboration with the Vrije Universiteit Amsterdam and published in the scientific journal BMJ Open.
Electronic health records contain data about care and health recorded by healthcare providers about their patients. These records contain many different types of information about the patient and the treatment. Creating multiple underlying relationships in these records results in new information about the patient with predictive value. Machine learning is a methodology that allows us to uncover these complex relationships from large amounts of data. This makes machine learning suitable for early detection of heart failure and other diseases.
Instant time savings
A machine learning model was developed specifically for the early prediction of heart failure in patients based on healthcare data from the GP office. We were able to identify patients at increased risk of heart failure one year before the moment the GP made the diagnosis. This is a significant time gain and secures the initiation of the next step in the care pathway: early screening of the patient.
Benefits of using GP data: many and upfront
We used data from Nivel Primary Care Database, which contains routinely recorded care data from 370 GP practices . This has two advantages: first, it provides us with a large amount of data, a prerequisite for meaningful use of the machine learning model we developed. Second, by using data already collected we were able to avoid additional burden on the patient and GP; we did not have to actively approach a patient and GP before making our predictions. This is an important note for future screening; however, this requires further validation of the model first.
About the research project
The Nivel conducted the study in collaboration with the Vrije Universiteit of Amsterdam.
We investigated the possibility of predicting heart failure one year before diagnosis, using the data of the electronic health records of patients over 70 years of age, collected from 2012 to 2019. These data include consultation information, medications, demographics, and lab values. As additional information we included the order in which the information was registered. For the analysis, we used three different machine learning algorithms: logistic regression, random forest and XGBoost.
The incidence of patients with heart failure in our study was similar to the incidence in practice. This makes it more likely that our results are translatable to practice.